Last updated on April 12, 2016
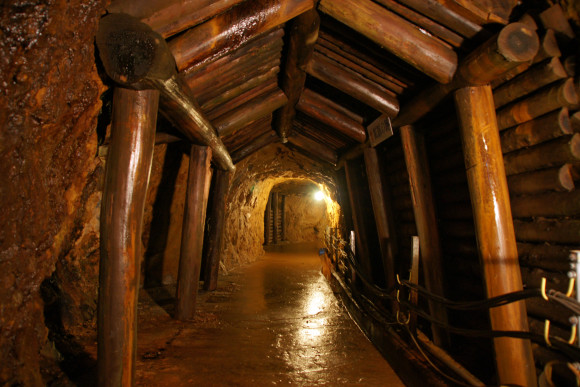
Last month, Ben Chapman and Don Schaffner, who host the Food Safety Talk podcast, discussed my January Gray Matter column in the New York Times in January, in which I discussed my work on farmers markets and food-borne illness.
Their discussion was even-handed, and Don (I think it was him; I listened to the segment only once, over a month ago) demonstrated a surprising understanding of the working paper culture in economics, wherein we circulate working papers well ahead of submitting for publication so as to make our work better in view of publishing it in better journals. But the one part which made my ears perk up was when Ben asked Don (or the other way around; again, it’s been a while since I listened) why my coauthors and I had looked at the relationship between farmers markets and all those seemingly irrelevant illnesses, and Don said (and I’m paraphrasing), “I don’t know, it looks like data mining.”
This made me conscious once again of the gap there exists between economics and other disciplines when it comes to empirical work. Bench scientists think papers in economics are much too long: “Why do you need to describe the data in so much detail?,” or “Why do you need all those tables that show the same thing over and over?”
Briefly, on why we need to describe the data in so much detail, much of the cleavage between economics and bench science comes from the fact that bench scientists deal exclusively with experimental data. In economics, however, for all this talk of field experiments and lab-in-the-field experiments, experimental data is still the exception and observational data the norm. And when you are dealing with observational data, chances are you are dealing with survey data, in which case you are almost surely dealing with responses provided by human beings whose answers are not always the most reliable. In such cases, it is helpful to explain where, when, and how the data were collected so your readers can tell whether there might be anything hinky going on with your results.
On why we need all those tables, that too has to do with the fact that much of economics deals with observational data. Unlike experimental data, which often allow for a simple comparison of means between treatment and control groups, observational data require one to slice the data in many different ways to make sure that a given finding is not spurious, and that the researchers have not cherry-picked their findings and reported the one specification in which what they wanted to find turned out to be there.
As such, all those tables of robustness checks are there to do the exact opposite of data mining. And as for why I look at seven different types of food-borne illness in my work on farmers markets and food-borne illness, that’s because when you find a positive relationship between, say, D and Y = Y_{1} + Y_{2} + … + Y_{7}, it makes sense to want to know whether that positive relationship comes from Y_{1}, Y_{2}, …, or Y_{7}, i.e., the different constituent parts of Y.
On the one hand, finding that none of the constituent parts of Y is associated with D would cast the initial finding that there is a relationship between D and Y in question. If I had found that outbreaks or cases of none of the top seven food-borne illnesses reported by the CDC were associated with the number of farmers markets, my main finding would have been pretty weak. On the other hand, finding that one ore more of the constituent parts of Y is associated with D is interesting in its own right. In my work, this means that knowing that the positive relationship between farmers markets and food-borne illness in general is due to a similar positive relationship between both (i) farmers markets and norovirus and (ii) farmers markets and campylobacter is interesting, since this can guide future research and policy making efforts.
That said, I completely get where Ben and Don were coming from with the comment that this looked like data mining. When you deal primarily (if not exclusively) with experimental data, looking at the relationship between your treatment variable and an increasing number of outcomes will eventually yield significance. Even if there is no causal relationship, you would expect significance at the 1, 5, or 10 percent significance level by looking at the relationship between your treatment and 100, 20, or 10 a priori unrelated outcomes. In that case, looking at more outcomes would indeed be data mining. That is why economists have recently started advocating for submitting a pre-analysis plan when doing experimental work, i.e., a document you submit before you begin collecting data in which you explain exactly which outcomes you will be looking at and how. (See here for a counterpoint that argues that pre-analysis plans are not always that useful, especially in case where one can do replication research.)