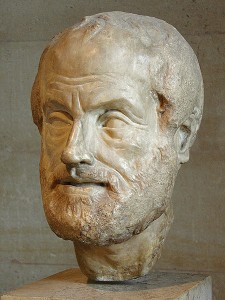
I was in Washington last month to discuss my work on food prices, in which I look at whether food prices cause social unrest, at an event whose goal was to discuss the link between climate change and conflict.
As many readers of this blog know, disentangling causal relationships from mere correlations is the goal of modern science, social or otherwise, and though it is easy to test whether two variables x and y are correlated, it is much more difficult to determine whether x causes y.
So while it is easy to test whether increases in the level of food prices are correlated with episodes of social unrest, it is much more difficult to determine whether food prices cause social unrest.
In my work, I try to do so by conditioning food prices on natural disasters. To make a long story short, if you believe that natural disasters only affect social unrest through food prices, this ensures that if there is a relationship between food prices and social unrest, that relationship is cleaned out of whatever variation which is not purely due to the relationship flowing from food prices to social unrest. In other words, this ensures that the estimated relationship between the two variables is causal. This technique is known as instrumental variables estimation.
Identifying Causal Relationships vs. Ruling Out All Other Causes
As with almost any other discussion of a social-scientific issue nowadays, the issue of causality came up during one of the discussions we had at that event in Washington. It was at that point that someone implied that it did not make sense to talk of causality by bringing up the following analogy: